Hello stranger, and welcome! 👋😊
I'm Rasmus Bååth, data scientist, engineering manager, father, husband, tinkerer,
tweaker, coffee brewer, tea steeper, and, occasionally, publisher of stuff I find
interesting down below👇
There are tons of well-known global indicators. We’ve all heard of gross
domestic product, life expectancy, rate of literacy, etc. But, ever
since I discovered
pinballmap.com, possibly
the world’s most comprehensive database of public pinball locations,
I’ve been thinking about a potential new global indicator: Public
Pinball Machines per Capita. Thanks to Pinball Map’s
well-documented
public API, this indicator
is now a reality!
Here’s how this was
put together (and just scroll to the bottom for a CSV file with this
indicator for all countries).
Upon discovering that the tiny town I live in has a pinball arcade with
over 40 tables (!), I got a bout of pinball fever. I fancy myself a
fairly accomplished video game player, but was disappointed to discover
that my ability to keep Mario alive didn’t translate to preventing the
pinball from draining. Assuming I just needed a bit of practice, I
downloaded
a virtual version of Fish
Tales — a fun,
fishing-based table from 1992 — and began practicing. Here’s the data
and quick analysis of how I improved over 100 games of Fish Tales.
(By the way, if you didn’t know, the hobbyist pinball emulation scene is
amazing. Almost every real pinball table from the last 70 years has
been painstakingly 3D-model by someone and is
available completely
for free, but completely not legally…)
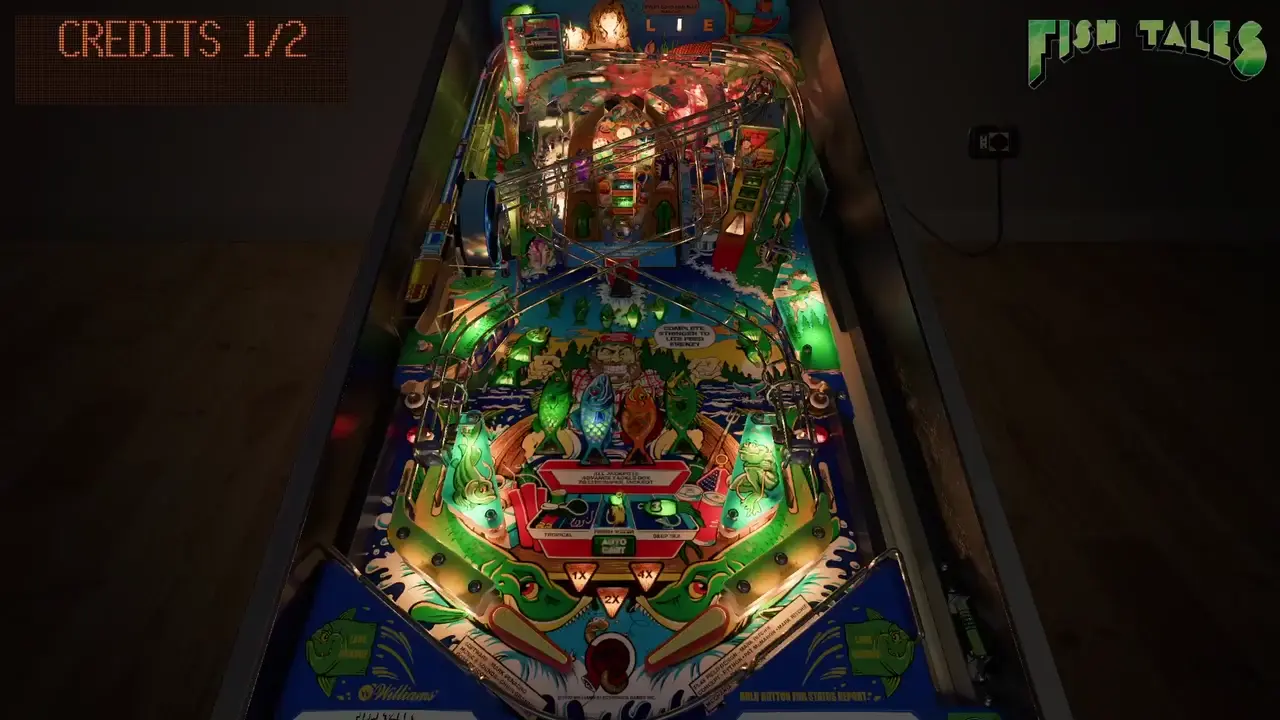
Five years ago I started a new role and I suddenly found myself, a
staunch R fan, having to code in Python on a daily basis. Working with
data, most of my Python work involved using
pandas
, the Python data frame library,
and initially I found it quite hard and clunky to use, being used to the
silky smooth API of R’s
tidyverse
. And
you know what? It still feels hard and clunky, even now, 5 years later!
But, what seems even harder, is explaining to “Python people” what they
are missing out on. From their perspective, pandas is this fantastic
tool that makes Data Science in Python possible. And it is a fantastic
tool, don’t get me wrong, but if you, like me, end up in many “pandas is
great, but…”-type discussions and are lacking clear examples to link to;
here’s a somewhat typical example of a simple analysis, built from the
ground up, that flows nicely in R and the tidyverse but that becomes
clunky and complicated using Python and pandas.
Let’s first step through a short analysis of purchases using R and the
tidyverse. After that we’ll see how the same solution using Python and
pandas compares.
Now that I’ve got my hands on
the source of the cake
dataset I knew I had to attempt to
bake the cake too. Here, the emphasis is on attempt, as there’s no way
I would be able to actually replicate
the elaborate and
cake-scientifically rigorous
recipe that Cook
followed in her thesis. Skipping things like beating the eggs exactly
“125 strokes with a rotary beater” or wrapping the grated chocolate “in
waxed paper, while white wrapping paper was used for the other
ingredients”, here’s my version of Cook’s Recipe C, the highest rated
cake recipe in the thesis:
~~ Frances E. Cook's best chocolate cake ~~
- 112 g butter (at room temperature, not straight from the fridge!)
- 225 g sugar
- ½ teaspoon vanilla, extract or sugar.
- ¼ teaspoon salt
- 96 g eggs, beaten (that would be two small eggs)
- 57 g dark chocolate (regular dark chocolate, not the 85% masochistic kind)
- 122 g milk (that is, ½ a cup)
- 150 g wheat flour
- 2½ teaspoon baking powder
1. In a bowl mix together the butter, sugar, vanilla, and salt
using a hand or stand mixer.
2. Add the eggs and continue mixing for another minute.
3. Melt the chocolate in a water bath or in a microwave oven.
Add it to the bowl and mix until it's uniformly incorporated.
4. Add the milk and mix some more.
5. In a separate bowl combine the flour and the baking powder.
Add it to the batter, while mixing, until it's all combined evenly.
6. To a "standard-sized" cake pan (around 22 cm/9 inches in diameter)
add a coating of butter and flour to avoid cake stickage.
7. Add the batter to the pan and bake in the middle of the oven
at 225°C (437°F) for 24 minutes.
Here’s now some notes, photos, and data on how the actual cake bake went
down.
In statistics, there are a number of classic datasets that pop up in examples, tutorials, etc. There’s
the infamous iris dataset (just type iris
in your nearest R prompt),
the Palmer penguins (the modern iris replacement),
the titanic dataset(s) (I hope you’re not a guy in 3rd class!), etc. While looking for a dataset to illustrate a simple hierarchical model I stumbled upon another one: The cake
dataset in
the lme4
package which is described as containing “data on the breakage angle of chocolate cakes made with three different recipes and baked at six different temperatures [as] presented in Cook (1938)”. For me, this raised a lot of questions: Why measure the breakage angle of chocolate cakes? Why was this data collected? And what were the recipes?
I assumed the answers to my questions would be found in Cook (1938) but, after a fair bit of flustered searching, I realized that this scholarly work, despite its obvious relevance to society, was nowhere to be found online. However, I managed to track down that there existed a hard copy at Iowa State University, accessible only to faculty staff.
The tl;dr: After receiving help from several kind people at Iowa State University, I received a scanned version of Frances E. Cook’s Master’s thesis, the source of the cake dataset. Here it is:
Cook, Frances E. (1938). Chocolate cake: I. Optimum baking temperature. (Master’s thesis, Iowa State College).
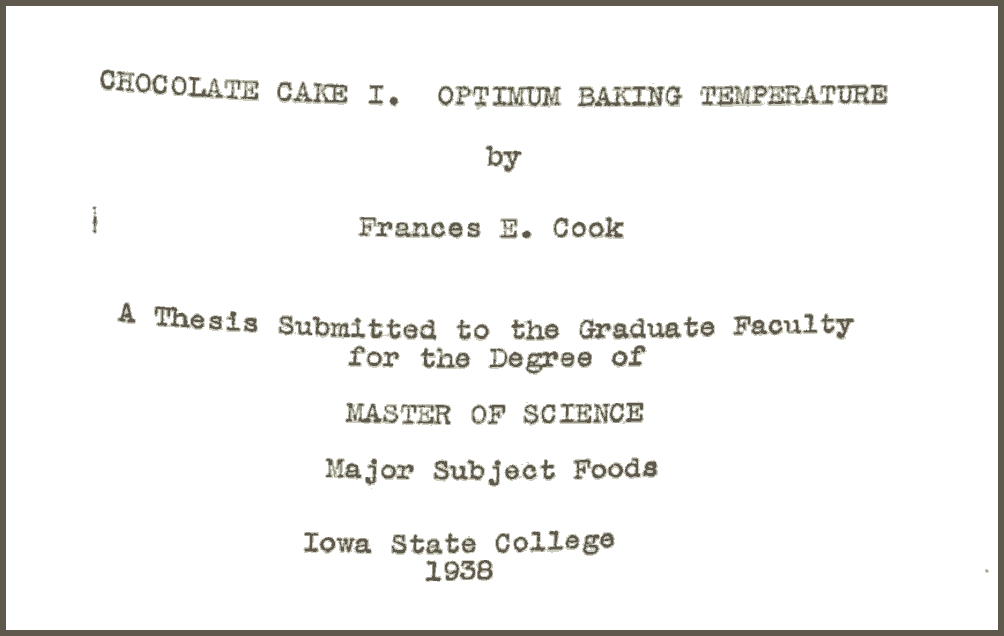
It contains it all, the background, the details, and the cake recipes! Here’s some more details on the cake dataset, how I got help finding its source, and, finally, the cake recipes.
If you know one thing about bubble sort, it’s that it’s a horrible sorting algorithm. But Bubble sort is great for one thing. Bubble sort has one good use case: Beer tasting. Let me explain:
I’ve now been in the industry long enough to know that meetings are often too long. No one likes to be in meetings, and the longer they are, the worse it is. Not only do I know most meetings are too long, but I also know exactly how long a meeting should be, at most! Let’s not delay it any further; here’s Rasmus’s maximum meeting length formula:
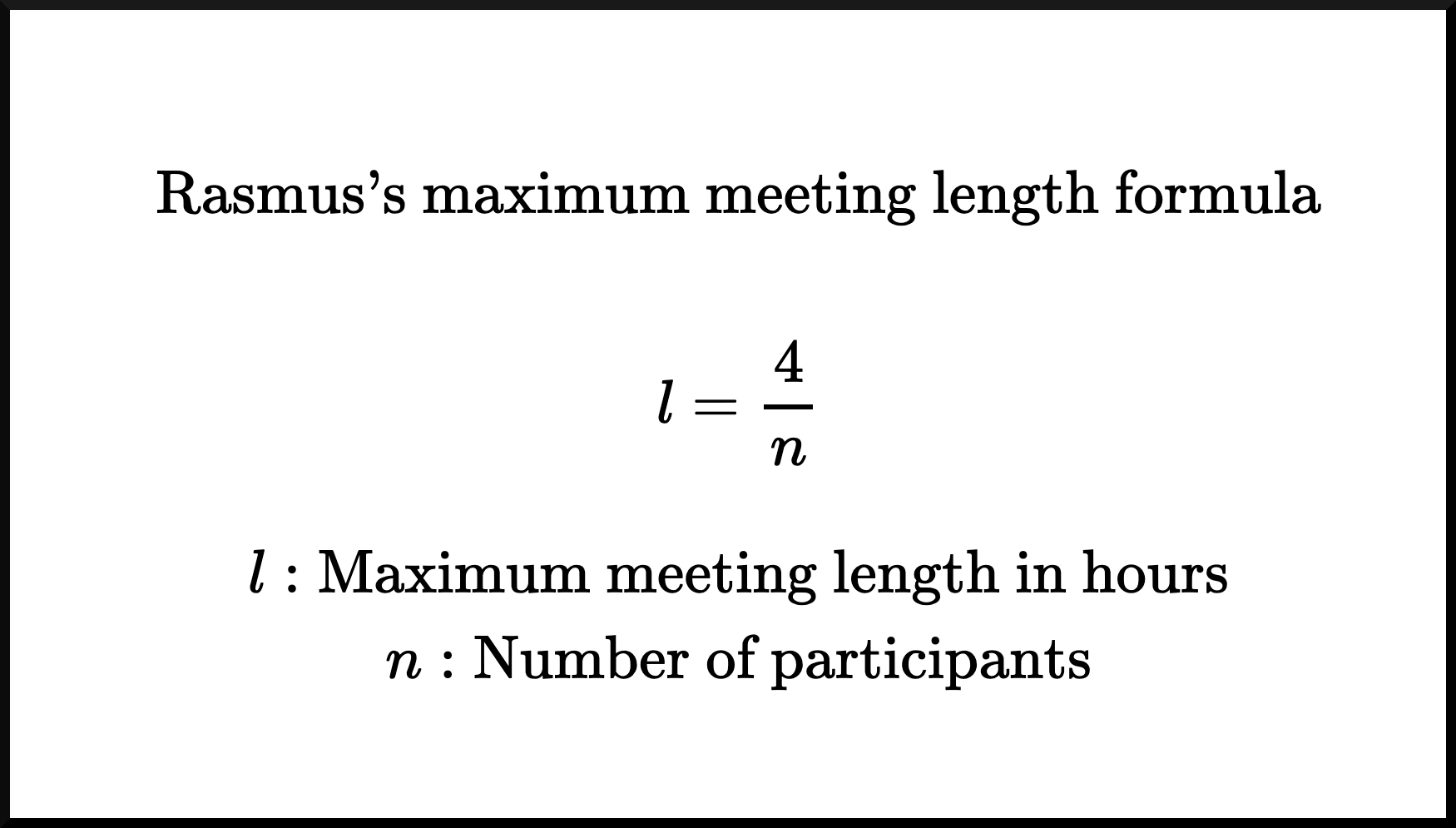
You can see that I’m confident about the correctness of this formula, as I’ve already branded it with my own name and rendered it in $\LaTeX$.
If that’s not enough to convince you, let me break it down further:
When I started working as a Data Scientist nearly ten years ago, the data science team I joined did something I found really strange at first: They had a single GitHub repo where they put all their “throwaway” code. An R script to produce some plots for a presentation, a Python notebook with a machine learning proof-of-concept, a bash script for cleaning some logs. It all went into the same repo. Initially, this felt sloppy to me, and sure, there are better ways to organize code, but I’ve come to learn that not having a single place for throwaway code in a team is far worse. Without a place for throwaway code, what’s going to happen is:
- Some ambitious person on the team will create a new GitHub repo for every single analysis/POC/thing they do, “swamping” the GitHub namespace.
- Some others will stow their code on the company wiki or drop it in the team Slack channel.
- But most people aren’t going to put it anywhere, and we all know that code “available on request” often isn’t available at all.
So, in all teams I’ve worked in, I’ve set up a GitHub repo that looks something like this:
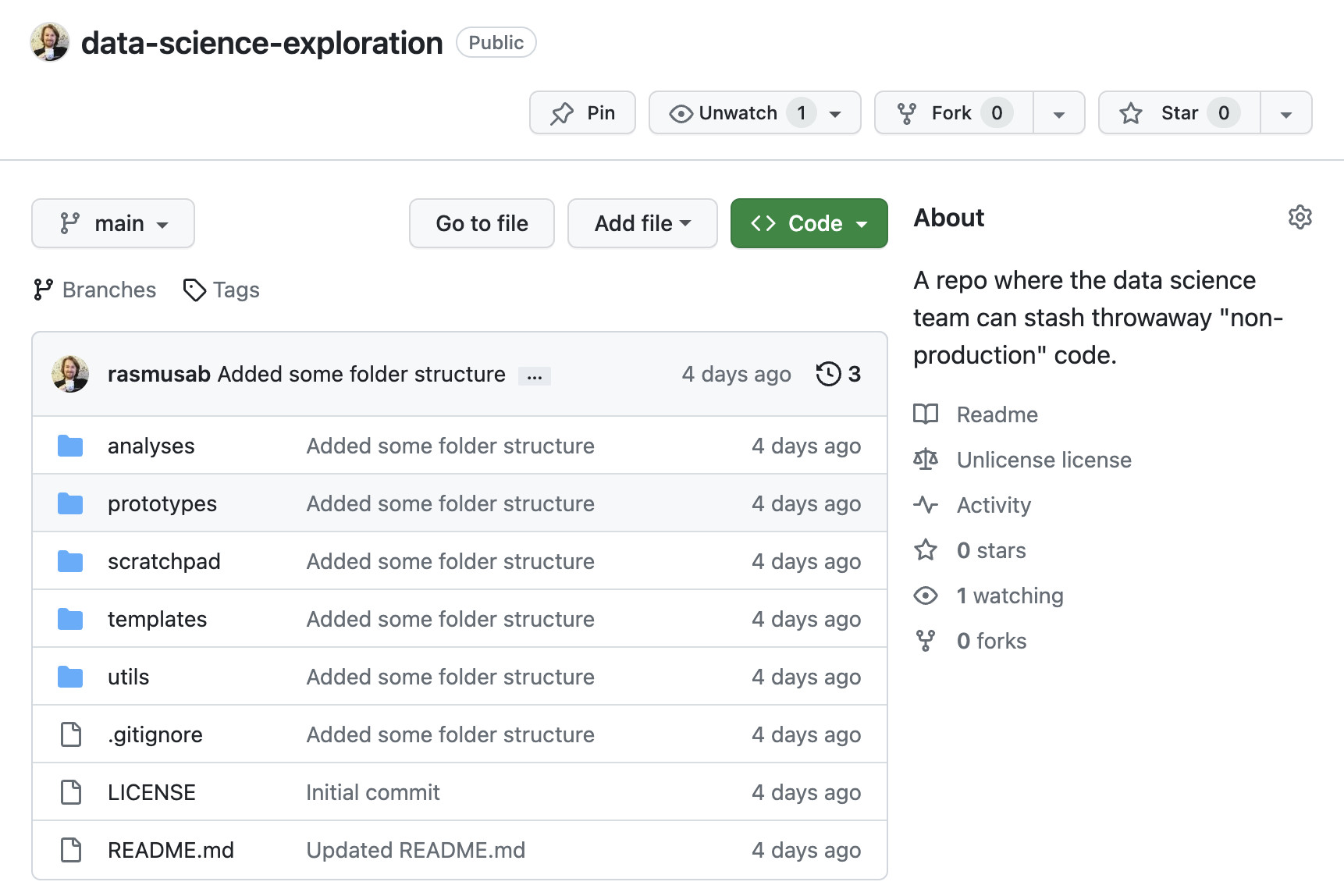
If you’ve ever looked at a Makefile
in a python or R repository chances are that it contained a collection of useful shell commands (make test
-> runs all the unit tests, make lint
-> runs automatic formatting and linting, etc.). That’s a perfectly good use of make
, and if that’s what you’re after then
here’s a good guide for how to set that up. However, the original reason why make
was made was to run shell commands, which might depend on other commands being run first, in the right order. In
1976 when Stuart Feldman created make
those shell commands were compiling C-programs, but nothing is stopping you from using make
to set up simple data pipelines instead. And there are a couple of good reasons why you would want to use make
for this purpose:
make
is everywhere. Well, maybe not on Windows (but it’s
easy to install), but on Linux and MacOS make
comes installed out of the box.
make
allows you to define pipelines that have multiple steps and complex dependencies (this needs to run before that, but after this, etc.), and figures out what step needs to be rerun and executes them in the correct order.
make
is language agnostic and allows you to mix pipelines with Python code, Jupyter notebooks, R code, shell scripts, etc.
Here I’ll give you a handy template and some tips for building a data pipeline using python and make
. But first, let’s look at an example data pipeline without make
.
The first thing I thought when I tried all the cool tools of the Year of the AI Revolution (aka 2022) was: OMG this is amazing, it’s the AI future that I never thought I would see. The second thing I thought was: OMG this is going to be used to spam the internet with so much bland auto-generated content.
I hate bland auto-generated content as much as the next person, but I was tempted by the forbidden fruit, I irresponsibly took a bite, and two short R scripts and a weekend later I’m now the not-so-proud owner of
officialcocktails.com: A completely auto-generated website with recipes, description, tips, images, etc. covering all the official International Bartenders Association cocktails.
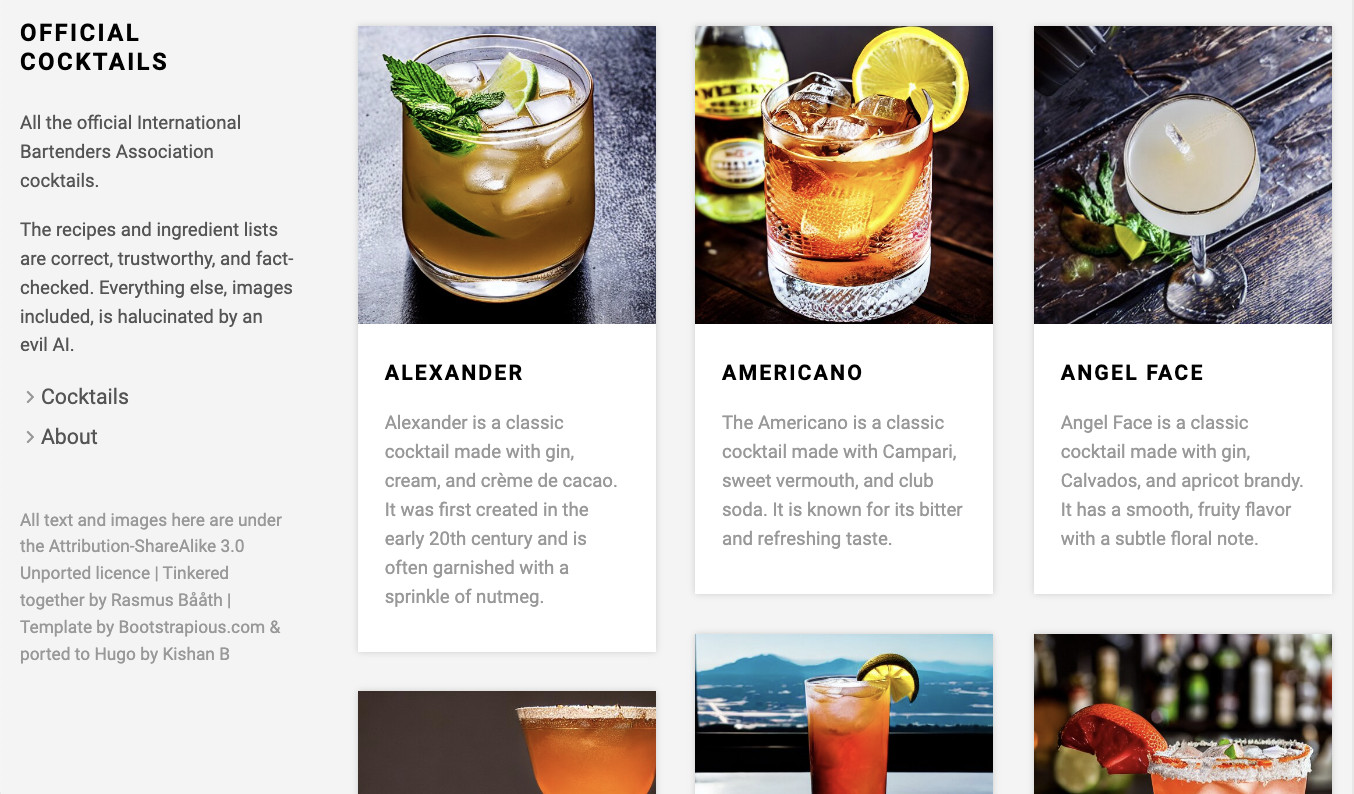
Here’s the quick recipe for how I whipped this up.